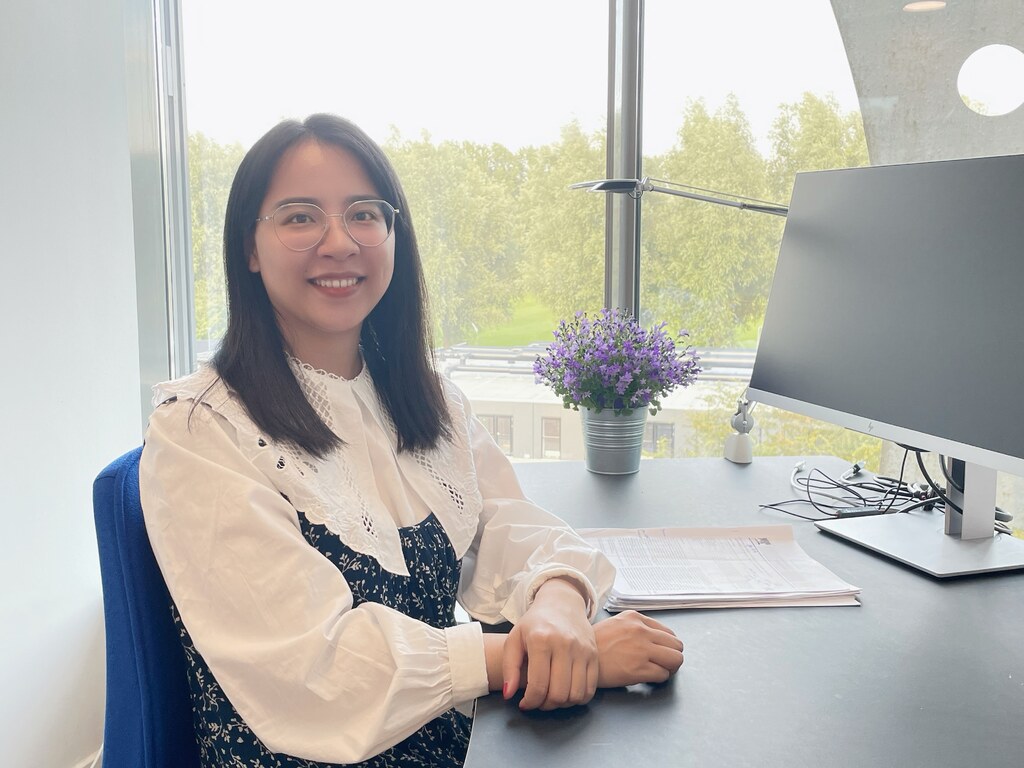
Transition to sustainable transport
As a prelude to Nordic Energy Challenge 2021, Nordic Energy Research presents the five selected participants in a portrait series. Meet Wu Chen. How have you previously worked with sustainable…
As a prelude to Nordic Energy Challenge 2021, Nordic Energy Research presents the five selected participants in a portrait series. Meet Wu Chen.
How have you previously worked with sustainable transport?
As a PhD student, I focused on sustainable transportation. My project “Towards sustainable transportation systems: Fleet dynamics, Resource Constraints, Emission pathways” concluded that a sustainable transition of the road transportation system requires high-quality mobility service with minimal resources and environmental impacts, which requires a systemic approach to analyzing the material and emission implications associated with different strategies to avoid burden shifting. I addressed these challenges and developed a dynamic, high resolution, fleet-based, and integrated modeling framework that linked the vehicle’s full life cycle with materials demand, energy use, and greenhouse gas emissions under different scenarios and pathways. I have used this modeling framework in four case studies on bicycles, passenger cars, and buses to demonstrate the interaction between fleet dynamics, resource constraints, and emission pathways in a sustainable road transportation transition.
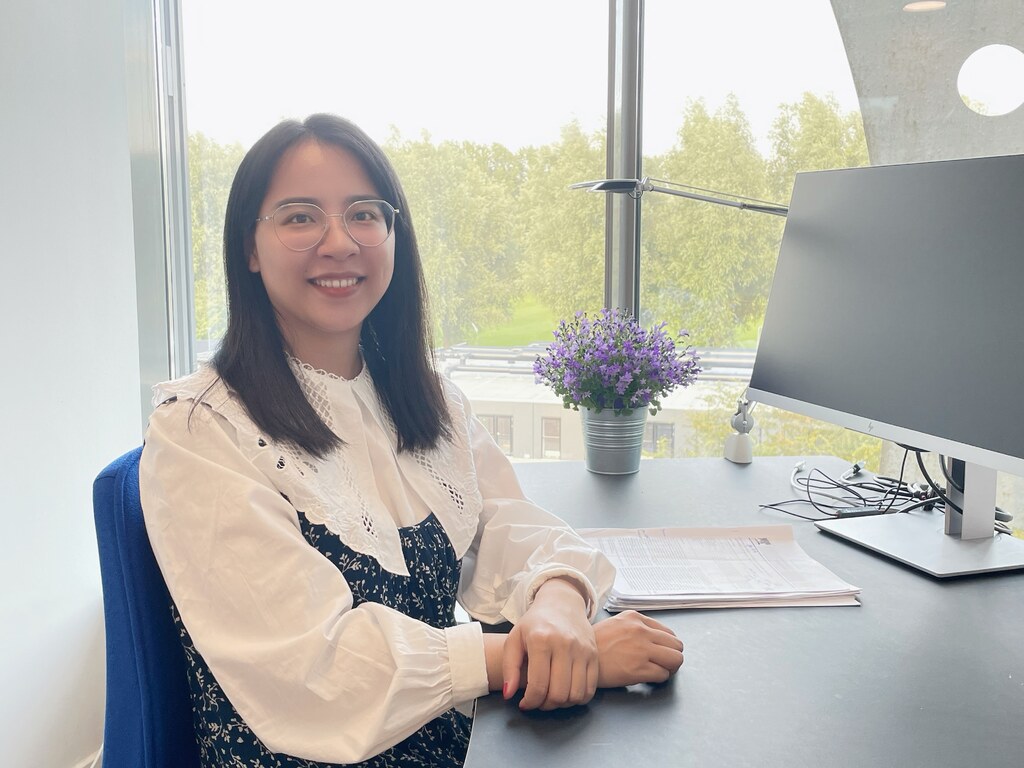
What is your idea about?
There are many challenges with current transportation systems: (1) poorly spatial-temporal characterization with more accurate data, (2) low stock efficiency, (3) missing a platform for traffic flow and electricity grid management. The digital transformation in the Nordics provides massive real-time spatiotemporal data to characterize the mobility behavior and improve the operational efficiency of a vehicle through mobility as a service platform.
The proposal aims to use the emerging data analytics techniques – machine learning and multiple available types of transportation data – such as mobile-phone GPS, remote sensing, and “point of interest” data, to open a new window for policymakers to see the potential of reducing vehicle kilometer, the system-wide transportation optimization, and associated emission reduction potential. The supervised and unsupervised machine learning methods could also help to integrate the transportation system into the energy system, creating synergies if available electric vehicles serve as energy storage systems. Furthermore, the big-data-driven mobility model with analytical method for machine learning could be extended to long-distance transport and freight logistics by improving the efficiency of freight consolidation.
How did you get the idea?
In recent years, artificial intelligence technologies have been gradually used to achieve sustainable development goals. Digital technology will gradually change everyday life around the world. A huge amount of data is created from different platforms, such as social media, mobile phones, computers, and vehicles. These real-time spatiotemporal data provides better opportunities to understand the transportation systems than before.
In my research I have read many scientific journals and found that these large-scale real-time data have been analyzed from machine learning models to evaluate the demand for gasoline and project the need for electric vehicle charging stations in the United States. These applications provide meaningful insights for future transport analysis in the digital transformation society.
How does your idea contribute to the Nordic region becoming CO2 neutral?
The Nordic countries are at the forefront of digital transformations and renewable energy technologies worldwide. By using machine learning methods together with the digital transformative society’s massive traffic data, the present and future mobility pattern can be better mapped. The detected mobility pattern efficiency (with fewer car emissions per passenger kilometer or per ton kilometer) could be further improved through routes optimization and consolidation using the machine learning methods.
Why is an event as the Nordic Energy Challenge important?
Nordic Energy Challenge provides a high-level platform for stakeholders, policymakers, and researchers to motivate innovative research, new ideas, and interesting perspectives. This year’s theme, sustainable transportation, is quite important for realizing a carbon-neutral and sustainable development due to the transport sector’s interactions with industry and the various energy sectors. Fleet dynamics, whether quantity, technology, or business model can have a huge impact on energy systems. The Nordic region sets ambitious carbon neutral goals and considers mobility as a service and electric vehicles as prioritized innovation. The reduced vehicle kilometers for driving sharing (a type of mobility service) can reduce the energy demand. More electric vehicles will reduce the direct emissions enormously, but also shift the burden to the electricity systems. The electric vehicles dramatically increasing need for electricity will challenge the electricity grid. The Nordic Energy Challenge aims to maximize Nordic added value and promote innovations. By researching and using technologies and business models with a low carbon content, the Nordic region will maintain its leading position worldwide.
Personal information
Name: Wu Chen
Education: PhD from Faculty of Engineering, University of Southern Denmark
Job: Postdoc, Institute Green Technology, University of Southern Denmark